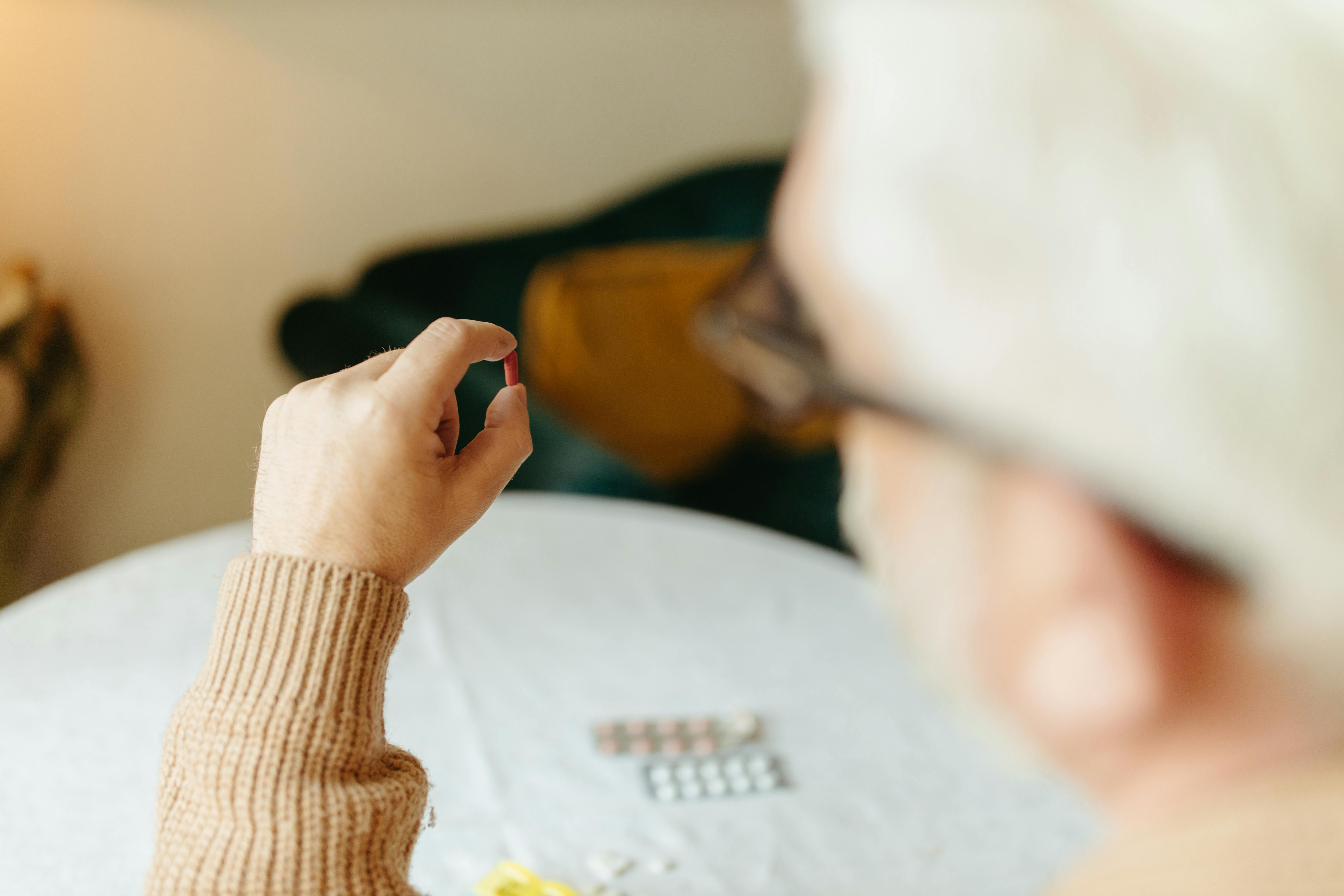
How to Effectively Calculate Error: Essential Methods for Accurate Results in 2025
Introduction to Error Calculation Techniques
Understanding how to effectively calculate error is crucial for achieving accurate results in various fields, including science, engineering, and data analysis. Errors can arise from many factors, and knowing how to quantify these errors is essential for improving accuracy and reliability. In this guide, we will explore the most essential methods of calculating error, helping you become adept at recognizing and rectifying inaccuracies in 2025 and beyond.
Types of Errors in Measurement
Errors in measurement can generally be categorized into three main types: systematic errors, random errors, and gross errors. **Systematic errors** are consistent inaccuracies that occur due to flaws in the measurement system, such as a miscalibrated scale. Conversely, **random errors** are unpredictable fluctuations in measurement that can stem from environmental factors or inherent limitations in the measuring device. **Gross errors** are notable deviations resulting from human mistakes, such as recording the wrong value or failing to account for external variables. Recognizing these types of errors enables you to select appropriate methods for calculating and correcting them effectively.
The Importance of Error Analysis
Conducting a comprehensive error analysis is essential to understand the reliability of your results. By identifying sources of error, researchers and analysts can refine their processes, enhance data integrity, and embrace strategies that mitigate risks of inaccuracies. Error analysis typically involves calculating metrics such as absolute error, relative error, and percentage error, all of which provide vital insights into the precision of measurements. This understanding is crucial for accurately interpreting research findings and harnessing meaningful conclusions in various applications.
Common Methods to Calculate Error
There are several methods to calculate error, each suited to specific circumstances. One popular method is the **absolute error**, which measures the difference between the measured value and the true value. This can be calculated using the formula:
Absolute Error = |Measured Value - True Value|. Another critical metric is the **relative error**, which expresses absolute error as a fraction or percentage of the true value. It can be represented mathematically as:
Relative Error = (Absolute Error / True Value) * 100%. Understanding these methods allows users to assess measurement accuracy effectively and take necessary corrective actions.
Advanced Techniques for Calculating Error
As the complexity of data analysis increases, advanced techniques for calculating error become paramount. Utilizing statistical methods can offer deeper insight into potential inaccuracies that might affect outcomes. Techniques such as regression analysis, standard deviation calculations, and confidence intervals can also help quantify multi-dimensional errors over an extensive data set, providing a holistic view of precision issues.
Regression Analysis and Error Estimation
Regression analysis is a powerful statistical tool that not only predicts outcomes but also helps calculate error by highlighting residuals—the deviations between observed and predicted values. By evaluating these residuals, one can compute the **Mean Squared Error (MSE)**, providing insight into the model's predictive accuracy. The formula for MSE is given by:
MSE = (1/n) * Σ(Observed - Predicted)^2. Incorporating regression analysis into your methodology can significantly enhance the accuracy of your results while offering robust insights for data-driven decision-making.
Using Standard Deviation for Error Assessment
Standard deviation is a critical measure in statistics that quantifies the amount of variation or dispersion in a set of values. By calculating the standard deviation of your measurements, you gauge how spread out the data points are regarding the mean value. The formula for the **standard deviation** is:
SD = √(Σ(x - μ)^2 / N), where μ represents the mean of the values. Recognizing the standard deviation of your calculations enables you to anticipate the degree of expected error and adjust experimental designs or data collection methods accordingly.
Confidence Intervals and Their Role in Error Measurement
Confidence intervals provide a statistical range in which the true value is likely to lie, offering critical context for measurement accuracy. They are constructed by taking the mean of your samples and adding/subtracting a margin of error derived from the standard deviation. This approach tells you the level of confidence you can have that the true value falls within this range and allows for superior decision-making and risk management in data interpretation.
Practical Examples of Error Calculation
Step-by-Step Calculation of Absolute and Relative Errors
Let’s walk through a practical example to solidify our understanding of error calculations. Suppose you measure the length of a table and find it to be 2.2 meters, but the actual length is 2.5 meters. To calculate the **absolute error**, you use:
Absolute Error = |2.2 - 2.5| = 0.3 meters. Next, to determine the **relative error**, you apply:
Relative Error = (0.3 / 2.5) * 100% = 12%. This method of tackling real-life measurements demonstrates a straightforward application of error calculations in daily contexts, allowing for greater understanding and oversight.
Case Study: Error Calculation in Scientific Experiments
Consider a scientific experiment where multiple trials are conducted to measure the effect of a chemical reaction. In values recorded across several trials, the original values are 3.6, 3.8, 4.0, 3.5, and 3.9 grams, whereas the true value is established at 4.0 grams. By applying the absolute and relative error calculations per trial, researchers can analyze which trials deviated significantly from the true value and could thus refine their methodologies in future experiments.
Adjustment of Methods Based on Error Findings
Upon calculating error, it becomes imperative to adjust measurement methods. If the calculated errors across several experiments indicate a systematic error, researchers may consider recalibrating their measuring equipment or employing different measurement techniques. This proactive approach to refining methodology can ensure that future measurements are accurate and reliable, which is crucial for maintaining scientific integrity and robust data.
Conclusion and Key Takeaways
In summary, mastering how to calculate error effectively is essential for ensuring accurate results in any analytical work. Familiarizing yourself with different types of errors and suitable calculation methods is key to enhancing measurement accuracy. Additionally, utilizing statistical techniques significantly improves the reliability of your outcomes. By following these guidelines and principles, you will be well-equipped to tackle the challenges of error calculation in 2025 and beyond.
FAQ
1. What is the difference between absolute error and relative error?
Absolute error is the direct difference between the measured value and the true value. In contrast, relative error contextualizes this absolute measure as a percentage of the true value, providing more insight into the error's significance in relation to the measurement itself. This distinction is crucial for evaluating the reliability of measurements and making informed decisions.
2. How can I reduce systematic errors in my measurements?
To reduce systematic errors, ensure your measuring tools are well-calibrated and maintained. Regular checks and calibrations against standard benchmarks, as well as following precise measurement protocols, can effectively minimize these errors. Designing experiments carefully to control environmental factors can also greatly enhance accuracy and reduce systematic discrepancies.
3. What role does standard deviation play in error measurement?
Standard deviation quantifies variations in datasets, providing an understanding of data dispersion about the mean value. This metric allows researchers to evaluate the precision of their measurements by indicating how closely data points cluster around the average—lower standard deviation implies more reliable results.
4. How can I interpret confidence intervals in error measurement?
Confidence intervals provide a statistical range within which the true value is expected to fall, enhancing your understanding of measurement reliability. A narrower confidence interval typically signifies higher accuracy, while a wider interval indicates increased uncertainty. This evaluation allows for better risk management and decision-making.
5. Why is error analysis important in scientific research?
Error analysis is critical for validating research findings, as it identifies inaccuracies and factors affecting measurement. By fostering a deeper understanding of potential errors, researchers can improve experimental design, enhance data integrity, and support reproducibility—elements paramount to scientific credibility and progress.